How good is Elon Musk at predicting the future? And what would it take to become an accurate predictor, yourself?
- Spencer Greenberg and Travis M.
- 56 minutes ago
- 17 min read

It's pretty useful to have a sense of what might happen in the future - or, at least not have false beliefs about it. This got us wondering: where are people getting their views about the future from? And how accurate is their information?
It occurred to us that perhaps the most listened-to forecaster in the world today is Elon Musk. He makes predictions regularly, and he has one of the biggest audiences in the world, with 218 million followers on Twitter/X (at time of writing). His predictions also fairly often get repeated by the media, amplifying their reach further. It's quite plausible that Musk's predictions about the future are heard, analyzed, and repeated more than those of any other person alive today.
That raises two questions, which we'll explore in detail in this article:
How accurate are Elon Musk's predictions about the future, actually?
How can a person (whether it's you, Elon Musk, or anyone else) become a more accurate predictor? The answer can help you become a better predictor on whatever topics matter to you.
We won’t be discussing Musk's entrepreneurship, companies, politics, controversies, or personality - we'll only be evaluating him as a forecaster, using data we've gathered by scouring the internet for as many of his predictions as we could locate. He'll serve as a case study to explore what it takes to make accurate predictions.
More broadly, we think that predictions matter. Making accurate predictions in your own life is a useful (and learnable) skill that has a lot of overlap with topics in critical thinking and rationality. And it's important, societally, that we know how much to trust predictions made by public figures. Accurate predictions help us make better decisions - at both an individual and societal level - whereas relying on inaccurate predictions leads us to make bad decisions.
If you want to skip the analysis of Musk's predictions, and just learn more about how to be a good forecaster, you can jump to the section titled “Part 2: How to Make Better Predictions”.
Key Takeaways 🧠 Musk is a prolific but unreliable forecaster. Our analysis of 43 time-based predictions found only about 16% came true on time. See the details below to dig into the methods and understand the limitations of this evaluation. ⚖️ Calibration matters when it comes to making predictions. To be a good predictor, your confidence and your accuracy should rise and fall together. 📚 Forecasting is a learnable skill. There’s a science to improving how well you predict the future. This article offers 5 tried-and-tested tips. 🛠️ There are lots of free tools for getting better at making predictions. With tools like our Nuanced Thinking Tool, Fatebook, and our Retrocaster assessment, you can practice being a better forecaster today! |
Part 1: Analyzing the Accuracy of Elon Musk’s Predictions
We searched the internet, high and low, trying to find predictions that Musk has made. We used three primary search methods:
Searching Twitter/X for posts by Musk containing predictions. This was done by searching for words associated with predictions (such as ‘predict’, ‘prediction’, ‘forecast’, and even the word ‘will’) and evaluating all the search results on a case-by-case basis to see whether they contained genuine predictions.
Google searches. We searched for stories about Musk’s predictions, as well as things like interviews in which predictions were made.
We also used OpenAI's Deep Research AI, asking it to find predictions he made, using this prompt:
“Find as many predictions that Elon Musk has made that you can find. Search in an unbiased way so that it's not biased towards predictions that came true or biased towards predictions that came false. We want to know about as many of his predictions as possible without bias in the search process.” |
Each of these results was then manually scrutinized to make sure it was a real prediction.
We think it is very unlikely that we managed to find all of Musk’s predictions. But we made a good faith attempt to find all the ones we could in a reasonable amount of time, and we attempted to do so without bias, so as to make the evaluation fair (more on this below). Should it turn out that we missed a meaningful number of predictions, we'll update our spreadsheet to account for them (so, if you know of any we didn't include, please let us know!).
We only included predictions that met the following criteria:
They contained a clear deadline (either explicit or implicit)
The predicted deadline had passed (so we know whether the prediction came true, or not)
Some predictions clearly and explicitly met both criteria, such as this one about Tesla cars in 2014:
”I'm confident that, in less than a year you'll be able to go from highway on ramp to highway exit without touching any controls.” |
Others did not have explicit deadlines, but we thought it fair to infer a rough implied deadline. For example, here’s a prediction made in 2012:
"However, China's real estate crisis will explode in a way that makes ours look puny. They can't hide it for much longer." |
It’s clear that the sentence ‘They can’t hide it for much longer’ implies that the ‘explosion’ will happen soon.
One important reason to eliminate predictions without deadlines is that, without a deadline, a prediction can never be false - it can only be either true or not true yet. This means that including such predictions in our data set could bias the sample.
In practice though, by eliminating predictions that did not have deadlines (explicit or implicit) or that were by their nature fundamentally unverifiable, we found that we eliminated only a small handful of predictions. Here are the predictions we eliminated:
Musk predictions with no deadline that came true | Musk predictions with no deadline that have not happened (or not happened yet) or that are unverifiable |
“I think that the internet is the superset of all media. One will see all media folding into the internet… it allows consumers to choose what they want to see and when they want to see it.” (Source) | “In the future, there will be no phones, just Neuralinks” (Source) |
“I think, uh, things are, things are very m–, definitely going to go into kind of autonomous, uh, locally autonomous drone warfare. That’s where it’s at. Where the future will be.” (Source) | “99% of cars will be electric and autonomous in the future. Manually-driven gasoline cars will be like riding a horse while using a flip phone. Still happens, but it’s rare.” (Source) |
It will cost ~$6bn to build a Hyperloop to take people to SF from LA in 30 minutes and the tickets will cost ~$20 per trip. (Source) | |
“Will be an option to add solar power that generates 15 miles per day, possibly more. Would love this to be self-powered. Adding fold out solar wings would generate 30 to 40 miles per day. Avg miles per day in US is 30.” (Source) Note: This one is ambiguous because although it is not a factory ‘option‘, there are companies not affiliated with Tesla that offer to install solar panels like this. | |
“There’s a one in billions chance we’re in base reality.” (Source) |
Additionally, our approach was to not include predictions that were trivial or things that almost everyone would predict (e.g., we wouldn't include "the sun will rise tomorrow") or where Musk could very easily make them come true (e.g., "Tesla will make a public announcement on Thursday" would not count since it would simply be Musk's decision whether that occurs).
Once we had gathered the predictions using our different search methods, we organized all of the ones that met our criteria into a spreadsheet. We also categorized each prediction by topic ("Tesla/cars", "SpaceX", "Society", "AI", "Boring Co.", "COVID", "Neuralink", "Twitter/X", "other") and carefully evaluated each prediction one-by-one, to see which had come true and which had not.
Obviously, determining whether any given statement counts as a prediction (and whether it has come true) is a task that requires judgment calls. As such, it’s inevitable that there will be some people whose judgment differs from our own. We tried to be careful and charitable, but you might still disagree with us. We expect that if you do disagree with us, it will be on only a very small number of cases, which ultimately won’t change the results very much. But why not see for yourself? You can look at all the claims we marked as predictions, in our public spreadsheet here. If you think we made any significant errors, please let us know so we can update the spreadsheet!
Here are the results:

Topic | Number of predictions | Number of true predictions | Percentage true predictions |
AI | 1 | 0 | 0% |
Boring company | 2 | 0 | 0% |
COVID | 2 | 0 | 0% |
Neuralink | 2 | 1 | 50% |
Other | 1 | 0 | 0% |
Society | 6 | 2 | 33% |
SpaceX | 10 | 2 | 20% |
Tesla or automotive | 17 | 2 | 12% |
Twitter/X | 2 | 0 | 0% |
Grand Total | 43 | 7 | 16.28% |
We found a total of 43 predictions that met our criteria, covering 9 domains. Musk’s overall success rate for these predictions was 16.28%.
When we split things up by domain, we see that there are some domains in which Musk did slightly better, for instance in his best category (which was Neuralink-related predictions) 1 out of 2 (i.e., 50% albeit of a very small sample) of predictions came true. The second most accurate category was Society, in which 2 out of 6 (i.e., 33%) of predictions came true.
How bad or good are these results?
Some people may say that it's unfair to judge Musk's predictions - that they aren't real predictions, they are just a form of marketing for his companies, to create hype, or a way to set deadlines to motivate his team. While it's possible that's true, we'd rather take Musk at face value, because he has explicitly told us how to interpret his predictions: in 2024, he tweeted that, for his time-based predictions, he “generally aim[s] for the 50% percentile date, which means that half [his] predictions will be late and half will be early.”

Our results show he is not achieving close to this desired level of accuracy. According to our analysis, only 16.28% of Musk’s time-based predictions come true by the time he says they will - much less than his stated goal of 50%.
Could it be that we somehow missed a lot of his good predictions, leading to a strong bias in our analysis? That’s always possible, we can't completely rule it out. But we don't think it's the case: we searched widely for predictions and approached the search in such a way as to attempt to reduce bias.
The difference between how accurate someone thinks their predictions are and how accurate they actually are points to an important idea in forecasting, known as "calibration". Let's take a moment to explore how it works.
Calibration Calibration is all about how well your confidence matches your actual results. A well-calibrated forecaster is right 90% of the time that they’re 90% confident, 50% of the time that they’re 50% confident, and so on. |
Note that calibration and accuracy are different measures of how good a forecaster is. A forecaster could be accurate (e.g., 90% of the time, what they claim comes true) but poorly calibrated (when they are more confident, their predictions are no more likely to come true than when they are less confident).
On the other hand, a forecaster could have medium accuracy (e.g., they are right only 50% of the time across all their predictions) but have good calibration (e.g., when they are 90% confident their predictions come true 90% of the time, when they are 10% confident their predictions are right 10% of the time).
Calibration is necessary for being a great forecaster, but it's not sufficient. That's because it's easy to be perfectly calibrated with 50% accuracy (e.g., on every yes/no prediction you can simply use a coin flip to determine whether to bet on yes or no - then you'll be 50% confident with each prediction and right 50% of the time, meaning you'll have 50% accuracy with perfect calibration).
The best forecasters are both calibrated AND accurate. Both are essential.
To unpack what being calibrated means a bit further (while simplifying matters a little), there are two broad ways to be uncalibrated. You can be:
Overconfident: This occurs when you systematically overestimate the chances that you are right - e.g., when you're 90% confident your predictions may only come true 70% of the time, and when you're 50% confident they may come true only 30% of the time.
Underconfident: This occurs when you systematically underestimate the chance that you are right - e.g., when you're 90% confident your predictions come true 99% of the time.
Most people suffer from overconfidence, but this is not a universal rule - some people are appropriately confident or underconfident.
Given that Musk says he aims for 50% of his predictions to come true by the time he says they will, we can infer that he has an average ~50% confidence that his predictions will come true on time.
A 50% average confidence may be the case for Musk, but for most people making public predictions we'd expect their average confidence to be meaningfully greater than 50%. In fact, we'd expect pretty much ALL predictions that forecasters publicly make (that are made in good faith and expressed confidently, without caveats) to come with more than 50% confidence. That's because having less than 50% confidence in something happening means thinking the thing is more likely to not happen. So, it would be misleading to publicly say that "X is going to happen" or "I predict that X" if you actually believed it with less than 50% confidence.
If we take Musks' claim about his own predictions at face value (that he aims to be right 50% of the time), then our data suggest that he is very poorly calibrated, and extremely overconfident. His predictions that he says have a 50% chance of coming true appear to come true only about 16% of the time.
That's his calibration. What's harder to evaluate is how good Musk's accuracy (16%) really is. While 16% seems like a very bad accuracy, that's not necessarily the case - to evaluate someone's accuracy you need to look at the difficulty of their predictions.
To understand why, consider archery as a metaphor. If someone hits their target in 16% of shots, we can't evaluate how good they are at archery unless we know how hard the shots they were taking were. If they were shooting arrows at far away drones while standing on the back of a galloping horse then 16% could be an incredible accuracy. Whereas if they are shooting a large target ten feet away then 16% could be a terrible result.
That means that being "accurate" will be domain dependent - in easy-to-predict domains you may need to be right 95% of the time to be considered "accurate", whereas in harder domains even being right 50% of the time may be excellent.
We recommend you checkout the spreadsheet of predictions for yourself to gauge how good or bad you think 16% accuracy is when predicting these sorts of topics.
Regardless of how good or bad you consider a 16% accuracy to be, if he's right only about 16% of the time then, in future, when you see that he's made a prediction about something, you shouldn't change your mind about it's likelihood very much. In contrast, if he was right 90% of the time, the fact that he's made a prediction would (if you were acting rationally) change your mind a lot more.
If you’d like to test your own calibration, you're welcome to try our free tool: Calibrate Your Judgment, which is a quiz designed to help you become adept at making well-calibrated judgments. It also tracks your progress over time so you can see how you improve! And there are thousands of potential questions it can ask you, to keep the tool fresh each time you use it.
The best arguments against what we're saying
Could we be wrong about Musk's predictions? There’s always a chance that most of Musk’s good predictions are harder to locate than his bad predictions, and even making significant attempts to use unbiased search strategies will leave most of his good predictions unfound. If this were the case, then our study would likely be unfairly biased against him.
For instance, maybe journalists only report when he is wrong, whereas when he is right they ignore it, leaving a much easier to find digital trail for his wrong predictions. This seems much more likely for his recent predictions than older ones, since mainstream journalists seem to be much more against him recently than they were in the past. While we tried to mitigate the potential for this issue by using multiple search methods, we can't be sure it didn't impact our results. This is also why we're very open to submissions of predictions of his that we've missed - we care about getting this right and avoiding bias as much as possible.
We released a preliminary version of this analysis a little while back, to help crowdsource predictions that we may have missed and learn about mistakes we may have made in our categorizing of results. Thousands of people saw the preliminary version, which did lead to a few updates to our spreadsheet, but did not change the results meaningfully. If you are aware of predictions we missed (that have an explicit or implicit time-based deadline), we’d like to hear about them! You can let us know by emailing us at info@clearerthinking.org and we will make periodic updates to the spreadsheet in light of submissions we receive. We request that if you plan to look for predictions Musk has made in order to submit them to us, that you aim to do so using a search strategy that isn't going to surface only failed predictions or only successful predictions.
Part 2: How to Make Better Predictions
So, the evidence we have suggests that Musk is not very good at making calibrated predictions. But he could learn to be better if he wanted - and so can you! In this section, we unpack 5 tips on how to improve your predictive abilities.
1. Think in probabilities
The best forecasters prefer not to say “That will happen” or “That won’t happen.” Instead, they give estimates as to how likely they think something is to happen. You’ll become a better forecaster if you get used to thinking this way. So, for instance, instead of thinking, “I know Jim will be late to the party,” you might think “I’m about 90% confident that Jim will be late to the party.” This is a powerful technique because it forces us to be precise, and to consider the possibility that we may be wrong.
When you’re trying to make a prediction, you’ll be considering evidence, but some evidence is weak and some is strong. You need to be able to adjust your confidence that something will happen, based on the strength of the evidence - so, if you get a little bit of evidence, you need to be able to adjust a little bit. If you get a lot of evidence, you need to be able to adjust a lot. This is hard to do without probabilities. Probabilities are flexible; you can bump them down or up as the evidence calls for.
If you want help with thinking this way, you can try our free, interactive Nuanced Thinking tool that will walk you through probabilistic thinking in more detail!
2. Break problems down
Break complex questions down into manageable parts. If you’re trying to predict whether Russia will pull out of Ukraine, that’s really tough to predict. But there are smaller and (at least somewhat) more tractable problems you can answer with more confidence that will then help you answer the bigger problem. By decomposing a prediction on a complex topic into a series of simpler ones you can improve your overall predictions on that complex topic.
The Drake Equation is a delightful example of this kind of thinking. You might wonder: how many alien civilizations are there in our galaxy that we could potentially communicate with? That’s an incredibly tough question to get any traction on by itself, but by breaking it down we can get some (very rough) ideas. The Drake Equation breaks this question down into seven smaller questions:
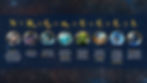
The number of civilizations in the Milky Way Galaxy with which communication might be possible =
The rate of formation of stars in the galaxy, multiplied by
The fraction of those stars with planetary systems, multiplied by
The number of planets per solar system with an environment suitable for life, multiplied by
The fraction of suitable planets on which life actually appears, multiplied by
The fraction of life-bearing planets on which intelligent life emerges, multiplied by
The fraction of those planets with intelligent life that develop interstellar communication, multiplied by,
The length of time such civilizations release detectable signals into space
Many of those smaller questions are still very difficult to answer, and proposed answers to the Drake equation vary by several degrees of magnitude, but breaking the problem down like this makes it much, much easier to handle (even though it's still difficult).
This kind of breakdown is also helpful because many predictions depend on multiple things happening. For example, if you're trying to figure out whether P will happen, and you realize that both A and B need to happen first, then thinking about the chances of A, and then considering the chances of B (if A does occur) provides critical information about the chance of P. Even if you can’t fully answer the big question, analyzing the smaller parts can help you narrow things down and give you traction on the larger thing you’re trying to predict.
3. Use reference classes
Let’s say you’re trying to estimate something really tough, like “How likely is it that the stock market will crash in the next 12 months?” That’s really hard. Nobody can know for sure. But what you can do is look at how often major market crashes have happened in each 12-month periods over the past 50 years. Suppose you find that a crash happened in about 10% of 12 month periods. That becomes your ‘base rate’ (the historical frequency of the event you’re trying to predict).
Even though this doesn’t tell you what will happen in the future, it gives you an evidence-based starting point. Now you can ask yourself “Do I think the next 12 months will be more likely to include a crash than usual?” And you can look for evidence for and against that hypothesis. That’s a much easier and more structured way to approach the problem. Without a base rate we're often merely guessing. With a base rate, we have a solid foundation to build on.
There’s evidence (discussed in the book Superforecasting: The Art and Science of Prediction, which we recommend reading if you want to get better at this) that using base rates like this helps people make better forecasts. Even simple historical data, if it’s relevant, can significantly improve your accuracy when predicting uncertain events.
4. Keeping track of your predictions
If you really want to get better, you have to keep track of how you’re doing. Indeed, this holds true for almost anything: if you’re not getting feedback, you’re going to learn slower than you otherwise could.
To this end, there’s a nerdy, useful, free tool called Fatebook that will help you keep track of your predictions. Clearer Thinking founder Spencer Greenberg uses this in his daily life to track how his personal predictions pan out and finds that it’s a great way to practice and learn.
Spencer has made 149 predictions on Fatebook so far, with an average confidence of 71%, and 67% of these predictions came true. Thankfully, as his confidence goes up his accuracy goes up too (a good sign for calibration). On the other hand, you can see from the graph of his confidence versus prediction accuracy (below) that he's a little bit overconfident, especially when he's 60%-70% confident (something he hopes to improve with further practice):

If you're interested in practicing making predictions, you may want to give Fatebook a try!
5. Seek disconfirming evidence
As human beings, our thinking is naturally prone to confirmation bias. This is a cognitive bias where we unconsciously favor information that supports our existing beliefs and dismiss or warp information that contradicts them. If you want to make better predictions, then you’ll want to counteract this bias by actively seeking disconfirming evidence. So, if you think something is going to happen, but you care about making accurate predictions, make sure you take some time to consider why it might not happen, and to seek evidence and arguments that contradict your current perspective.
This point might seem obvious, but it’s shocking just how many people don’t do it. We’ve written about this before as one of the most foundational and biggest-impact things you can do to think more rationally. Spencer also reports that AI LLMs work great for this: you can tell them something you believe and ask them to "give the strongest counter arguments, and provide the strongest scientific evidence, against this point of view."
Conclusion
Predicting the future (also known as forecasting) is a difficult skill. Elon Musk is one of the most prominent people currently engaging in it, but our study of his predictions found that he was right only ~16% of the time when he gave a deadline (despite apparently having ~50% confidence). It’s likely that he is very overconfident about his time-based predictions.
If you want to get better at making predictions yourself, you can remember the five tips we’ve outlined above:
Think in probabilities
Break problems down
Use reference classes
Keep track of your predictions
Seek disconfirming evidence
If you want to read more about how to improve your predictive abilities, we highly recommend Philip Tetlock’s book Superforecasting: The Art and Science of Prediction.
And, if you want a quick test of your forecasting abilities, you can try our free quiz to see whether you can predict the direction of various recent global trends (and get results right away) - from population sizes to CO2 emissions and more!